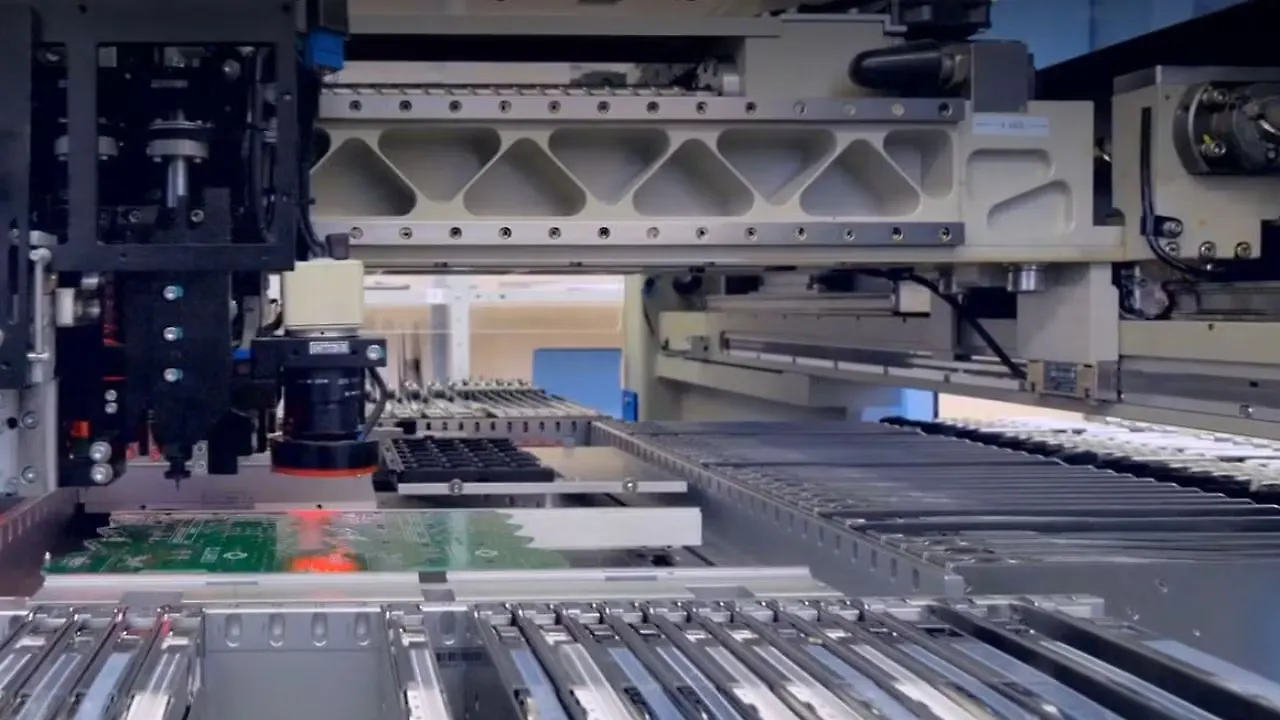
Infiswift Technologies is upbeat about its business in India, the US, and Japan.
“Our technology and development centre in Pune has been a huge success. It has allowed us to provide around-the-clock responsiveness to our customers and partners,” Lillu Srinivasan, Managing Director, Infiswift Technologies (India) told Mobility Outlook.
The company offers project-ready artificial intelligence to real-world challenges and helps manufacturing enterprises in their Industry 4.0 journey. According to her, the company’s IoT+AI Fabric can seamlessly integrate with clients’ SCADA systems, sensor networks and other data sources.
Expertise in customising the Fabric to a specific manufacturing plant offers the flexibility to run AI-driven applications on the cloud or — for added security — on the plant network. Srinivasan said the AI and data science algorithms process vast amounts of data generated by IoT devices. This analysis is crucial for predictive maintenance, quality control, remote monitoring and control and process optimisation.
IoT sensors and cameras capture data such as measurements, images and other quality-related information during production. Its computer vision and deep learning techniques process this data to identify defects, anomalies, or deviations from quality standards. Faulty products can be detected early, reducing wastage and ensuring high-quality output.
Asset Tracking
Infiswift’s PULSE, a specialised asset tracking product, helps optimise the supply chain by using beacons, RFID tags, GPS etc providing real-time visibility into the supply chain and improving inventory levels, demand forecasting and logistics. This includes tracking the location and condition of goods during work-in-progress stages and transit.
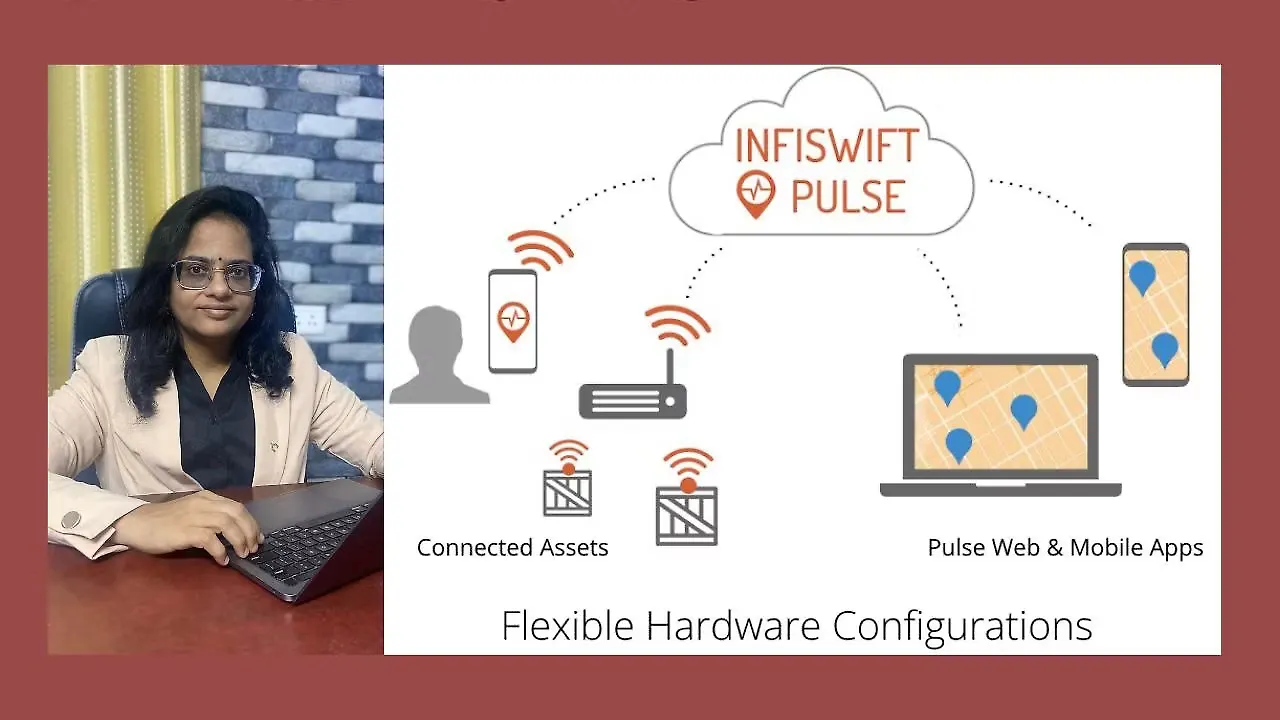
According to Srinivasan, customised machine learning models can predict demand fluctuations more accurately, enabling companies to adjust production schedules dynamically. The AI-driven supply chain optimisation models can be tailored to consider specific suppliers, transportation routes, and manufacturing locations. These can dynamically adapt to changing market conditions and supplier capabilities.
However, flawless production is critical while enhancing machine availability. These issues are addressed by the IoT sensors that collect real-time data from machines. “We can customise predictive maintenance algorithms to specific machines and components using this data,” she said.
AI algorithms can predict spare parts likely to be needed and maintain a readily available inventory to reduce the time required to replace faulty components. IoT-Enabled remote monitoring can also help diagnose the issues quickly and resolve them.
To support OEMs making multiple models in the same line where feeding the right parts is key, PULSE maps the parts required for the models. It attaches RFID tags or barcodes to each part for the operator to choose the right parts for the model.
Mitigating Bottlenecks
Srinivasan said unsupervised ML algorithms can understand assembly lines to track the cyclical operating patterns for every machine. “Once we capture this data, our AI algorithms identify production line level and sub-machine level bottlenecks and raise alerts. This empowers the operators to address relevant issues and improve the plant's throughput,” she said.
Taking a cue from a case study, Infiswift devised an AI-driven web application that helped operators trace back to the settings that cause those bottlenecks and fix them. The application also quantifies and prioritises these obstacles so operators can know in real-time which is the weakest link to be improved to maximise the RoI.
How does Infiswift help manufacturing companies reduce their time-to-market while making products flawless, safer and sustainable? Srinivasan said the company’s capabilities in the data science domain helped implement a customer churn prediction algorithm based on the need. Since it indicates how many existing clients’ customers are leaving their business, it positively impacts the revenue streams.
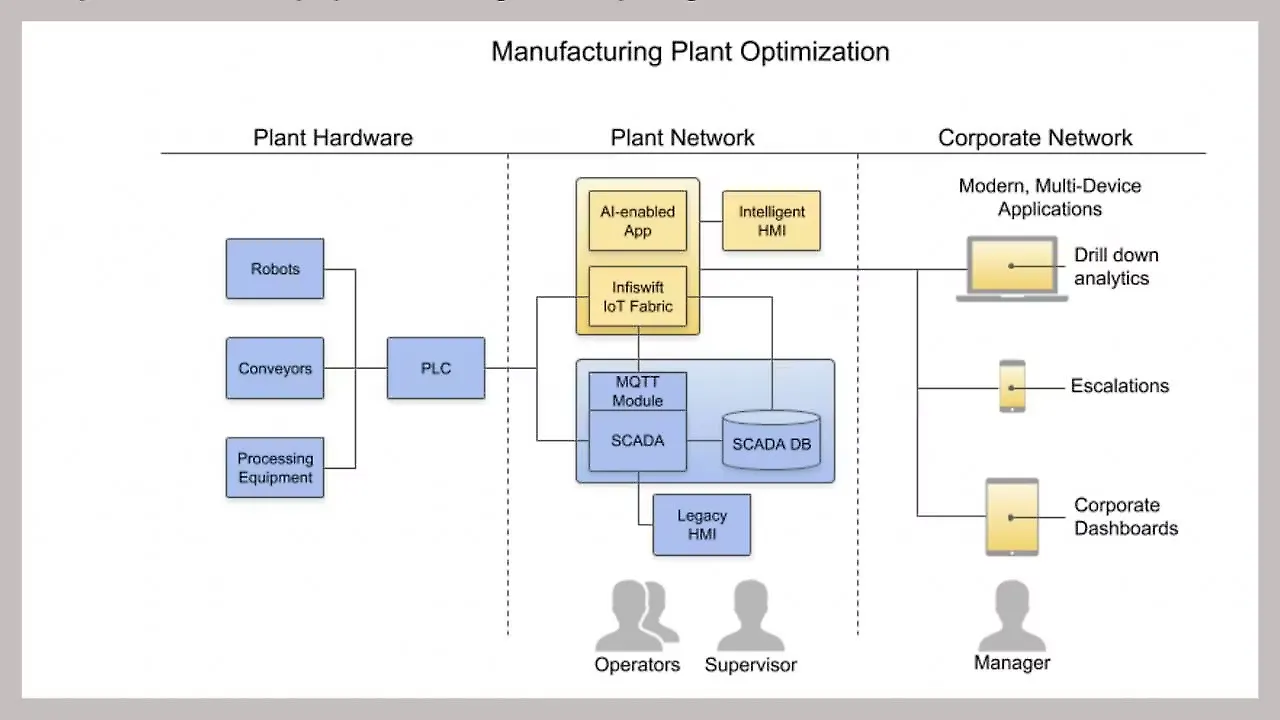
While churn rates track lost customers, growth rates track new ones. By comparing and analysing, these metrics tell how much the business is growing over time. “Project complexity and timelines vary, but in general, our projects show proof of value within 4-8 weeks and positive RoI within the first 6-12 months,” she added.
Electric Vehicles
For EVs, the company has developed battery management and optimisation AI algorithms. These predict the health and maintenance requirements of the battery, ensuring optimal performance and reducing downtime. It can also optimise charging and discharging patterns based on user behaviour, energy costs, extending battery life and enhancing range.
Capturing data is easier than crunching them. Infiswift supports its customers with a wide range of data science solutions that help realise value at different stages of the data science process. “One of our customers was a small startup, for whom we built the data pipelines from scratch to help them collect the required data for building ML algorithms later. On the other hand, several of our customers already have a large data lake and ask us for help solving business problems,” elaborated Srinivasan.
The company used its AI fabric to develop customised data science solutions. A large oil & gas services company in the US had 20,000 PDF files with diagrams and tables. They had collected this data over decades and wanted to convert this unstructured dataset to a more structured format. These could then be stored in a database and a dashboard built using the structured data.
Hidden Patterns
“Our AI digitisation algorithms can find hidden patterns in unstructured PDF and excel files, allowing us to extract data precisely in the required output formats. This allowed the customer to realise the value of decades worth of data,” said Srinivasan.
From her point of view, there are many opportunities for innovation in the automotive industry and Infiswift is involved in some like smart inventory solutions. Conventional RFID tracking has been deployed in both warehouses and shop floors for decades.
Pain points resulting from rapid changes or non-linear workflows have traditionally not resulted in significant RoI. However, adding AI and multiple inputs to existing tracking systems can solve these inventory problems.
In software, the concept of microservices has introduced a whole new way of thinking about systems and the company sees this happening in real life manufacturing settings. “Where a person used to cycle lubricants in equipment based on a set schedule, we see opportunities to create intelligent lubrication systems based on sensor feedback. This reduces the human cost to maintaining equipment and extending its life of the equipment,” signed off Srinivasan.
Also Read:
Capgemini, Munich Technical University To Deploy AI In EV Batteries